the Creative Commons Attribution 4.0 License.
the Creative Commons Attribution 4.0 License.
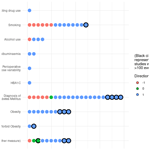
Systematic review of risk prediction studies in bone and joint infection: are modifiable prognostic factors useful in predicting recurrence?
Andrew Hotchen
Martin A. McNally
Jamie Hartmann-Boyce
Matthew Scarborough
Gary Collins
Background: Classification systems for orthopaedic infection include patient health status, but there is no consensus about which comorbidities affect prognosis. Modifiable factors including substance use, glycaemic control, malnutrition and obesity may predict post-operative recovery from infection. Aim: This systematic review aimed (1) to critically appraise clinical prediction models for individual prognosis following surgical treatment for orthopaedic infection where an implant is not retained; (2) to understand the usefulness of modifiable prognostic factors for predicting treatment success. Methods: EMBASE and MEDLINE databases were searched for clinical prediction and prognostic studies in adults with orthopaedic infections. Infection recurrence or re-infection after at least 6 months was the primary outcome. The estimated odds ratios for the primary outcome in participants with modifiable prognostic factors were extracted and the direction of the effect reported. Results: Thirty-five retrospective prognostic cohort studies of 92 693 patients were included, of which two reported clinical prediction models. No studies were at low risk of bias, and no externally validated prediction models were identified. Most focused on prosthetic joint infection. A positive association was reported between body mass index and infection recurrence in 19 of 22 studies, similarly in 8 of 14 studies reporting smoking history and 3 of 4 studies reporting alcohol intake. Glycaemic control and malnutrition were rarely considered. Conclusion: Modifiable aspects of patient health appear to predict outcomes after surgery for orthopaedic infection. There is a need to understand which factors may have a causal effect. Development and validation of clinical prediction models that include participant health status will facilitate treatment decisions for orthopaedic infections.
- Article
(469 KB) - Full-text XML
-
Supplement
(112 KB) - BibTeX
- EndNote
Risk assessment plays a pivotal role in clinical decision-making. Clinical prediction models combine prognostic factors into an equation to estimate the probability of a patient experiencing a health outcome in the future. Models can also predict response to a treatment, exacerbation of a condition, or an adverse event such as mortality (Grant, 2018). Clinical prediction models may be used to decide the setting of care (NICE QS110, 2016), whether or not a diagnostic test should be used (Stiell, 1992) or a particular treatment should be offered (NICE CG181, 2016), and to communicate risk around clinical decisions (Wishart et al., 2010).
Clinical prediction models can be used to estimate the success of bone and joint infection treatment according to a patient's baseline health. This has been applied following debridement, antibiotics and implant retention (DAIR) for prosthetic joint infection (PJI) (Duffy et al., 2018; Wouthuyzen-Bakker et al., 2019).
Existing classification systems for orthopaedic infections differ in what health factors they include. The McPherson classification of PJI uses the number of comorbidities to stratify host health; however, why particular comorbidities were chosen is unclear (McPherson, 2002). The validation of host classification in the McPherson system detected no association between host status and treatment outcome in 50 patients. Host status was associated with the “number of surgical complications”, which included urinary retention, thrombocytopaenia and non-allergic antimicrobial reactions ranked equally with stroke, septic shock, respiratory failure and death.
The BACH stratification tool for long bone osteomyelitis has recently been developed and validated, categorising distribution of infection within a bone, antibiotic options, the management of the soft tissue required for wound closure, and host status. Host status is classified as favourable (H1) or unfavourable (H2), with some suggested comorbidities conferring H2 class. Discrepancies were observed in how H2 was interpreted by clinicians (Hotchen et al., 2019). The Cierny–Mader classification of long bone osteomyelitis divides adverse host factors into local and systemic compromising conditions (Mader et al., 1997). It specifies “tobacco abuse, i.e. > 2 packs d−1” as the cut-off for local compromise but does not describe how this was derived. Phrases such as “major vessel compromise” were not defined and may be open to interpretation.
Modifiable prognostic factors affecting health, such as malnutrition, blood glucose control, smoking and alcohol use, can affect healing and immunity. Interventions addressing these factors can improve outcomes after surgery (Norman et al., 2008; Thomsen et al., 2014; Barr et al., 2016; Hopkins et al., 2017). Prognostic modelling studies could help identify whether these modifiable prognostic factors predict successful treatment for bone and joint infections. If accurate, validated clinical prediction models that include comorbidities and modifiable prognostic factors already exist, they could inform the “host status” section of classification systems such as BACH. This would help to (a) select treatment for bone and joint infections; (b) discuss with patients the likelihood of a successful treatment outcome as part of valid informed consent; (c) provide a benchmark for expected rates of treatment success; and (d) select participants for investigation of treatment methods.
Important outcomes for patients considering orthopaedic surgery include pain, mobility and independence, fear (including of sepsis and severe illness), sleep quality, work, social function, and the burden of treatment (Trickett et al., 2012; Baumhauer et al., 2013; Moore et al., 2015). Generic patient-reported outcome measures (PROMS) contain some of these measures and may be particularly useful when consensus criteria for infection eradication are not available (Lipsky et al., 2004; Baumhauer et al., 2013; Diaz-Ledezma et al., 2013; Metsemakers et al., 2018).
The development, reporting and validation of clinical prediction models have been extensively reviewed. Guidance is available for publication and assessing reporting quality (Hayden et al., 2013; Moons et al., 2014, 2015; Collins et al., 2015; Grant et al., 2018; Wolff et al., 2019). TRIPOD guides the reporting of studies describing the creation and validation of clinical prediction models (Collins et al., 2015; Moons et al., 2015). Different methodological assumptions apply to prediction modelling and analysis for causal inference.
The primary objective of this study was to systematically review and critically appraise clinical prediction models that included patient comorbidities (particularly modifiable prognostic factors), developed for the prognosis of surgically treated musculoskeletal infection. We aimed to find models that included substance use (at least smoking and alcohol intake), hyperglycaemia, malnutrition, and obesity.
An additional objective was to systematically review prognostic studies that did not fit the definition of clinical prediction modelling to identify the direction of association between potentially modifiable prognostic factors and treatment success. The aim of this analysis was to identify the usefulness of modifiable factors for prognostic modelling, rather than causal inference.
A systematic review (PROSPERO CRD42020177814) was conducted to evaluate published studies reporting epidemiological or prognostic modelling of orthopaedic infection recurrence after curative surgery (including removal of infected implants and debridement) in adults.
EMBASE and MEDLINE databases were searched from inception to July 2020. Reference lists from studies identified during the review were searched. Search terms for diagnoses included synonyms for spondylodiscitis, osteomyelitis, fracture-related infection, and prosthetic joint infection. Terms for surgical treatment and outcome were included. Synonyms for variables relating to smoking, alcohol use, hyperglycaemia, malnutrition, and obesity were included, chosen based on preliminary searches in November 2018. Search strings are presented in the Supplement.
The following studies were excluded: case-control studies unadjusted for population outcome risk; patients treated without surgery or without implant removal; children < 18 years old; and those that did not include follow-up of at least 6 months.
The main outcome was recurrence of orthopaedic infection or new orthopaedic infection at the same anatomic site, defined using any criteria, including composite outcomes. This is referred to as “treatment failure”. PROMS were considered a secondary treatment outcome.
Studies were identified as risk prediction modelling studies if they reported a multivariable (two or more predictors) risk prediction model for treatment failure. Additionally, the aims, statistical modelling methods, model interpretation, intended use, and validation reported were assessed.
Data extracted included the study design, participant selection and loss, sample size, predictor selection and measurement; outcome incidence, definition and ascertainment; and modelling considerations. The latter included missing data handling, the choice of statistical model, whether assumptions were violated, and the handling of competing outcomes such as participant death or limb amputation. For clinical prediction modelling, model shrinkage for overoptimism, model calibration and discrimination and validation were recorded.
For clinical prediction studies, applicability and risk of bias were assessed using the PROBAST tool. For epidemiologic studies, domains from the QUIPS tool and CHARMS checklist were used to ascertain study relevance and stratify risk of bias from low to high across methodological domains (Hayden et al., 2013; Moons et al., 2014, 2015; Collins et al., 2015; Wolff et al., 2019). Appropriateness of sample size was assessed according to the number of listed predictors specified in the methods, or, if this was not available, predictors listed in univariable analysis. The minimum appropriate sample size was estimated using the pmsampsize package for R, assuming an estimated R2 of 0.25 (selected to be generous) and parameters from the study report (Ensor et al., 2019). Assuming an estimated average orthopaedic infection recurrence rate of 20 % at least 12 months following treatment, the maximum attainable Cox–Snell R2 is 0.63.
Data were extracted from studies by two researchers in parallel (Maria Dudareva, Andrew Hotchen). Risk of bias was assessed across domains independently. Disagreements were resolved by consensus with supervision from a third researcher if required (Gary Collins).
Due to the anticipated heterogeneity of studies following a preliminary search in November 2018, guidance for synthesis without meta-analysis (SWIM) was followed for summary measures for prognostic factors of interest (Campbell et al., 2020).
The direction of association for each prognostic variable was determined. This was defined as positive (point estimate of odds ratio of treatment failure > 1 for participants with the prognostic factor), negative (point estimate of odds ratio of treatment failure < 1 for participants with the prognostic factor), or null (estimated odds ratio equal to 1). Adjusted values were preferentially recorded, if this included the prognostic factor of interest. Unadjusted odds ratios, if not reported, were estimated from baseline characteristics or published datasets.
Analyses were performed using R version 3.6.3 (R Core Team, 2020).
Figure 1 describes the selection and inclusion of studies in this systematic review.
In total, 35 studies met eligibility criteria and were included, involving 92 693 participants recruited between 1987 and 2018. All studies were retrospective analyses of participants receiving treatment in secondary and tertiary centres. The geographical distribution of reported studies included 18 from the USA, 3 from Taiwan, 2 each from the UK, Germany, France and China, and 1 each from Canada, Brazil, Spain, Italy, South Korea and Denmark.
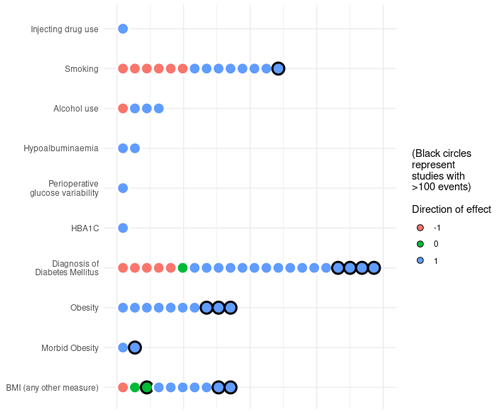
Figure 2Direction of association for grouped variables representing prognostic factors of interest identified from included studies. Each circle represents the direction of association for one variable in one study. Note that all studies were assessed at a high risk of bias.
Table 1 describes characteristics of included participants and their treatment. No studies performed multivariable modelling using PROMS, and only one study investigated the association between a prognostic factor and PROMS (Watts et al., 2014). Twenty-five studies included a multivariable analysis of treatment outcomes, while nine studies reported univariable analyses only. No studies published a model equation, though this was estimated from Kheir et al. (2018).
Statistical synthesis was not undertaken as all studies were assessed to be at high risk of bias, predominantly due to lack of information on how risk factors were measured and blinding between risk factor and outcome measurement (Hayden et al., 2013). Studies did not include the same prognostic factors of interest in adjusted analyses. Sample size was assessed for each study, and model overfitting resulting from the inclusion of too many prognostic variables contributed to the assessment of risk of bias (Moons et al., 2014).
Two studies fit the definition of risk prediction modelling studies (Sabry et al., 2014; Kheir et al., 2017). Neither study performed internal or external validation, nor was an external validation of these risk prediction models identified during this systematic review. Both studies were assessed against the PROBAST tool to have an overall high risk of bias (Table 2).
Table 2Risk of bias assessment for included risk prediction modelling studies according to the PROBAST tool (Wolff et al., 2019).
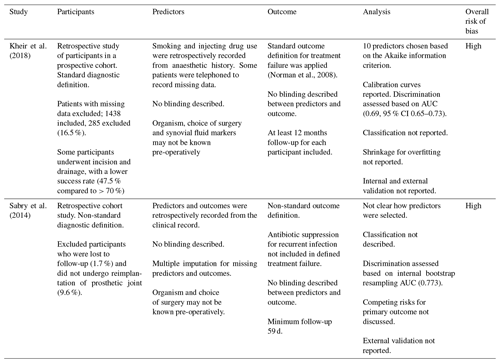
Kheir et al. (2018) presented a logistic regression model for treatment failure as a risk calculator based on points for baseline prognostic factors, following surgery for PJI. They included body mass index (BMI) and ever smoking in the prognostic calculator, as well as a number of non-modifiable factors. The largest estimated odds ratios of treatment failure for dichotomous variables were conferred by treatment with irrigation and debridement rather than implant revision (OR 2.48) and a history of myocardial infarction (OR 1.57). For every unit increase in BMI, the odds of treatment failure were estimated to increase 1.02-fold. For participants who had ever smoked, the odds of treatment failure were estimated to increase 1.2-fold.
Sabry et al. (2014) created a nomogram, based on a logistic regression model, for predicting treatment failure following two-stage revision arthroplasty for knee PJI. The prognostic variables included BMI, but adjusted odds ratios for the variables of interest were not reported.
Of 25 studies that reported multivariable statistical analyses, 20 did not have an adequate sample size to model the number of prognostic factors, according to the proportion of participants with the outcome and assuming R2=0.25 (Ensor et al., 2019). In 30 of 35 studies that included univariable prognostic modelling, fewer than 10 outcome events were included per prognostic variable of interest. In 14 studies, the number of prognostic variables described was equal to, or more than, the total number of participants experiencing the treatment outcome of interest.
A summary of the direction of association reported for potentially modifiable prognostic factors and related variables is shown in Fig. 2. One study reported unadjusted and adjusted odds ratios (ORs) for treatment failure in study participants with a history of injecting drug use (Jhan et al., 2017). No studies identified the association of nutritional status with the risk of treatment failure for bone and joint infection. Pre-operative albumin level, a surrogate marker used to assess nutritional status, was included in three studies, of which two studies reported a negative association between measured serum albumin and treatment failure. Two studies reported variables relating to glycaemic control (peri-operative blood glucose variability and HbA1C) (Barshes et al., 2016; S. H. Wang et al., 2019).
A greater proportion of larger studies, with more than 100 participants with the primary outcome, reported higher odds of treatment failure (positive direction of association) for smoking, a diagnosis of diabetes mellitus, and BMI when compared to smaller studies. The ratio of studies reporting a positive direction of association was greatest for glycaemic control and HbA1C (100 %, 2/2), albumin (100 %, 2/2) and BMI-related variables (86 %, 19/22), followed by alcohol intake (75 %, 3/4) and smoking (57 %, 8/14). One study reported a positive direction of association for injecting drug use (100 %, 1/1). This is summarised in Fig. 2.
Many prognostic studies reported the results of a hypothesis test of the direction and magnitude of the association between modifiable prognostic factors and treatment failure following surgery. The ratio of studies reporting a significant (P< 0.05) positive association was greatest for albumin (67 %, 2/3) and variables relating to BMI (42 %, 11/26), followed by alcohol intake variables (25 %, 2/8), diabetes diagnosis (20 %, 6/30), smoking (17 %, 4/24), glycaemic control (0/3) and injecting drug use (0/4).
Figure 3 shows unadjusted and adjusted odds ratios for treatment failure after surgery for participants with the modifiable prognostic factors of interest.
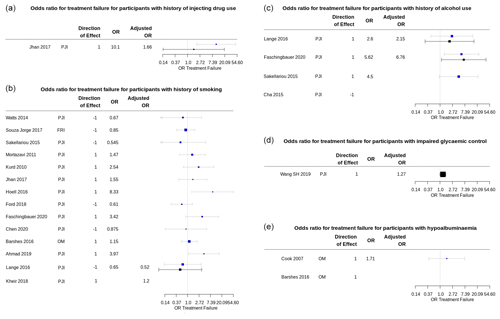
Figure 3Summary plots of the odds ratio (OR) for treatment failure in participants with a modifiable risk factor of interest reported in included prognostic studies. Univariable ORs are shown in blue; multivariable (adjusted) ORs are shown in black. Plots produced using package forestplot for R (Gordon, 2020). (a) Univariable and adjusted ORs for treatment failure for participants with a history of injecting drug use. The adjusted OR from Jhan et al. (2017) is for Cox proportional hazard regression analysis for treatment failure, adjusted for BMI, liver cirrhosis, microbiology investigations, the presence of a sinus tract, repeated surgical debridement, and operating time > 4 h. (b) Univariable and adjusted OR for treatment failure for participants with a history of smoking. Adjusted OR from Lange et al. (2016) is for treatment failure at 12 months in all study participants, adjusted for age, sex, American Society for Anaesthesiology (ASA) score, diagnosis of diabetes mellitus, BMI, and alcohol use, using logistic regression modelling from the published data. Confidence intervals for adjusted OR in Kheir et al. (2018), calculated from the risk prediction model-scoring equation, were not reported. (c) Univariable and adjusted ORs for treatment failure for participants with a history of alcohol use or dependence. Adjusted OR from Lange et al. (2016) is for treatment failure at 12 months in all study participants, adjusted for age, sex, ASA score, diagnosis of diabetes mellitus, BMI, and smoking, using logistic regression modelling from the published data. Adjusted OR from Faschingbauer et al. (2020) was adjusted for surgical approach (two-stage revision), additional revision between first- and second-stage surgery, and number of prior surgical procedures. (d) Adjusted OR for peri-operative blood glucose variability reported in S. H. Wang et al. (2019) was adjusted for “all confounders”, which is understood to include age, sex, BMI, joint involvement, Charlson comorbidity index, diagnosis of diabetes mellitus, rheumatic disease, index surgery (primary or revision), debridement and irrigation before spacer placement, and spacer exchange, as reported in the study methods. (e) Univariable OR for treatment failure for participants with hypoalbuminemia (serum albumin 2.2 to 3.0 g L−1) reported in Cook et al. (2007).
The identification and selection of participants were reported in 21 of 35 studies. Study setting and recruitment dates were reported in all studies. Study sample size was often inadequate relative to the number of prognostic factors investigated. This contributed to prognostic model overfitting, and thus most studies were considered at a high risk of bias. Participant exclusion following enrolment was often reported, contributing to bias in some studies. For example, a participant who died of severe infection that may have been related to treatment failure was excluded from the analysis (Cha, 2015). Study follow-up duration was well described, but loss to follow-up was up to 17 % and may have been higher in participants who had not experienced treatment failure (Kheir et al., 2017).
No studies described the measurement of prognostic factors in sufficient detail to allow replication. The source, definition, independence, and particularly timing of the prognostic factor were not specified. This could have been remedied if study protocols had been published. Additionally, few studies described the handling of missing values; only one study reported using multiple imputation (Sabry et al., 2014).
This systematic review identified prognostic studies aiming to predict the outcome of surgery for bone and joint infection that included modifiable prognostic factors. Few studies measured nutrition, peri-operative glycaemic control, or substance use for prognosis.
The two clinical prediction modelling studies had a high risk of bias according to independent review by two investigators using the PROBAST tool. Furthermore, the overall risk of bias of the other prognostic studies identified, using the QUIPS tool, was also moderate or high. The studies suggest that modifiable factors, including smoking, glycaemic control, and alcohol intake, predict higher odds of treatment failure. The prognostic value from these factors appeared to be outweighed by others, including diagnosed cardiac, hepatic or renal failure, the number and history of prior revision surgery for prosthetic joint infection, the surgical approach, and soft tissue coverage. No clinical prediction studies were identified that included participants with osteomyelitis or fracture-related infection (FRI).
Modelling prognosis in orthopaedic infection is complicated. Firstly, the treatment pathway may be difficult to standardise. This is illustrated by Anderson et al. (2018), who predicted successful treatment for patients with total knee arthroplasty infection that required extensor mechanism reconstruction, and by Barton et al. (2019), describing attrition in participants intending to receive two-stage revision hip or knee arthroplasty. In the first study, six participants did not start the intended treatment, and those who did had between 1 and 14 surgical procedures. In the second study, only 68 % of patients completed the intended treatment. The modes of treatment failure were functionally different and hence described as separate outcomes in the study report. Complex treatment pathways make it particularly challenging to select an a priori outcome for patients treated for orthopaedic infection. Different definitions of recurrence made it difficult to compare studies.
Treatment decisions are not independent of prognostic factors for treatment failure. Competing risks must be accounted for in prognostic studies. Mortality, amputation and long-term antimicrobial suppression, which were often not defined as treatment failure, are competing risks. Several studies reported a greater number of participant deaths than treatment failures (Chen et al., 2015; Russell and Tsang, 2020). In one study, participants did not undergo reimplantation surgery based on a prognostic factor and so were excluded from analysis (Q. Wang et al., 2019).
Without an explicit definition of how and when a prognostic factor was recorded, its use in risk prediction will not allow an accurate estimate of prognosis. “Smoking” may refer to a diagnostic code in the secondary care record, a note on an anaesthetic chart (Kheir et al., 2017), directly asking a patient, or confirming with carbon monoxide measurement. It may refer to smoking at any time in the past (Kheir et al., 2017), a particular minimum pack-year history (Barshes et al., 2016), pre-operative smoking (Barton et al., 2019), or ongoing smoking after surgery. The timing and dose are important – quitting 4 weeks before surgery appears to be associated with improved healing and a lower risk of primary osteomyelitis (Truntzer et al., 2014). Only one study reported the timing of a prognostic factor recording at least 12 months before the outcome (Son et al., 2017). The heterogeneity in OR of treatment failure for participants with a history of smoking may reflect these differences.
Limitations of the review process include language restriction due to the databases included (though studies published in German and French were reviewed), limitations of the search strategy, and amalgamation of orthopaedic infections affecting differing patient populations and carrying differing prognoses. Additionally, it is possible that some risk prediction modelling studies have been classified as prognostic studies. It can be challenging to infer from published reports whether a study is aiming at prognostic modelling or epidemiology, due to the lack of clarity in the term “risk factor” and the similar statistical methods used in both types of study. It is important that studies state the underlying assumptions of their modelling approach and describe whether the aim is prediction or causal inference (Schooling and Jones, 2018).
The modifiable prognostic factors considered in this systematic review are complex variables that may not have a linear relationship with prognosis and may not be independent. The association of social determinants of health with prognosis, access to healthcare, and the choice of treatments may be relevant to the external validity of prognostic models in different patient groups. Some studies reported health insurance as a surrogate marker for socio-economic status, but this was not included in reported clinical prediction models (Son et al., 2017; Barton et al., 2019; Q. Wang et al., 2019). Barshes et al. (2016) was the only study to assess participants' housing status and found a positive association between homelessness and treatment failure (Barshes et al., 2016).
Finally, independent external validation of prognostic models benefits from the publication of model equations to enable assessment of their calibration and discrimination in new populations. Neither of the risk prediction modelling studies included in this review published the prognostic model equation, so it was estimated from available data (Sabry et al., 2014; Kheir et al., 2017). Improvement in the quality of prognostic study reporting in orthopaedic infection will aid shared decision-making with patients prior to major surgery.
Data were manually extracted from published data in studies referenced in this review. R packages (open access) used in the presentation of the extracted data are referenced. Statistical techniques used to synthesise data are described in study methods and more specifically in figure legends to facilitate reproduction of these results.
The supplement related to this article is available online at: https://doi.org/10.5194/jbji-6-257-2021-supplement.
MD, JHB and GC developed the idea for the study. MD and AH extracted and analysed data with supervision by JHB, MM, MS and GC. MD, MM, JHB, MS and GC wrote and edited versions of the manuscript. All the authors agreed to submit the manuscript for publication.
The authors declare that they have no conflict of interest.
This study was funded by NIHR Doctoral Research Fellowship grant 300249. We are grateful to Paula Dhiman for advice about prognostic study classification.
This research has been supported by the National Institute for Health Research (grant no. 300249).
This paper was edited by Antonia Chen and reviewed by two anonymous referees.
Ahmad, S. S., Orlik, L., Ahmad, S. J. S., Albers, C. E., Siebenrock, K. A., and Klenke, F. M.: Obesity and smoking predict the results of two-stage exchange in septic revision hip arthroplasty: a cohort study, Orthop. Traumatol., 105, 467–471, https://doi.org/10.1016/j.otsr.2019.01.006, 2019.
Anderson, L. A., Culp, B. M., Della Valle, C. J., Gililland, J. M., Meneghini, R. M., Browne, J. A., and Springer, B. D.: High failure rates of concomitant periprosthetic joint infection and extensor mechanism disruption, J. Arthroplasty, 33, 1879–1883, https://doi.org/10.1016/j.arth.2018.01.022, 2018.
Barr, T., Girke, T., Sureshchandra, S., Nguyen, C., Grant, K., and Messaoudi, I.: Alcohol consumption modulates host defence in rhesus macaques by altering gene expression in circulating leukocytes, J. Immunol., 196, 185–195, https://doi.org/10.4049/jimmunol.1501527, 2016.
Barshes, N. R., Mindru, C., Ashong, C., Rodriguez-Barradas, M., and Trautner, B. W.: Treatment failure and leg amputation among patients with foot osteomyelitis, Int. J. Lower Extr. Wound., 15, 303–312, https://doi.org/10.1177/1534734616661058, 2016.
Barton, C. B., Wang, D. L., An, Q., Brown, T. S., Callaghan, J. J., and Otero, J. E.: Two-stage exchange arthroplasty for periprosthetic joint infection following total hip or knee arthroplasty is associated with high attrition rate and mortality, J Arthroplasty, 35, 1384–1389, https://doi.org/10.1016/j.arth.2019.12.005, 2019.
Baumhauer, J. F., McIntosh, S., and Rechtine, G.: Age and sex differences between patient and physician-derived outcome measures in the foot and ankle, J. Bone Joint Surg. Am., 95, 209–214, https://doi.org/10.2106/JBJS.K.01467, 2013.
Bejon, P., Berendt, A., Atkins, B. L., Green, N., Parry, H., Masters, S., McLardy-Smith, P., Gundle, R., and Byren, I.: Two-stage revision for prosthetic joint infection, predictors of outcome and the role of reimplantation microbiology, J. Antimicrob. Chemother., 65, 569–575, https://doi.org/10.1093/jac/dkp469, 2010.
Campbell, M., McKenzkie, J., Sowden, A., Katikireddi, S. V., Brennan, S. E., Ellis, S., Hartmann-Boyce, J., Ryan, R., Shepperd, S., Thomas, J., Welch, V., and Thomson, H.: Synthesis without meta-analysis (SwiM) in systematic reviews: reporting guideline, BMJ, 368, l6890, https://doi.org/10.1136/bmj.l6890, 2020.
Cancienne, J. M., Werner, B. C., Bolarinwa, S. A., and Browne, J. A.: Removal of an infected total hip arthroplasty: risk factors for repeat debridement, long-term spacer retention, and mortality, J. Arthroplasty, 32, 2519–2522, https://doi.org/10.1016/j.arth.2017.03.018, 2017.
Cancienne, J. M., Granadillo, V. A., Patel, K. J., Werner, B. C., and Browne, J. A.: Risk factors for repeat debridement, spacer retention, amputation, arthrodesis, and mortality after removal of an infected total knee arthroplasty with spacer placement, J. Arthroplasty, 33, 515–520, https://doi.org/10.1016/j.arth.2017.08.037, 2018.
Carrega, G., Casalino-Finocchio, G., Cavagnaro, L., Felli, L., Riccio, G., and Burastero, G.: Long-term outcome of prosthetic joint infections treated with two-stage revision, Acta Orthop. Belg., 86, 10–16, 2020.
CDC: NHSN Patient Safety Component Manual 2020, Chapter 17: Surveillance Definitions, available at: https://www.cdc.gov/nhsn/PDFs/pscManual/17pscNosInfDef_current.pdf, last access: June 2020.
Cha, M. S., Cho, S. H., Kim, D. H., Yoon, H. K., Cho, H. S., Lee, D. Y., Lee, S. H., and Hwang, S. C.: Two-stage total knee arthroplasty for prosthetic joint infection, Knee Surg. Relat. Res., 27, 82–89, https://doi.org/10.5792/ksrr.2015.27.2.82, 2015.
Chen, S. Y., Hu, C. C., Chen, C. C., Chang, Y. H., and Hsieh, P. H.: Two-stage revision arthroplasty for periprosthetic hip infection: mean follow-up of ten years, BioMed Res. Int., 2015, 345475, https://doi.org/10.1155/2015/345475, 2015.
Cochran, A. R., Ong, K. L., Lau, E., Mont, M. A., and Malkani, A. L.: Risk of reinfection after treatment of infected total knee arthroplasty, J. Arthroplasty, 31, 156–161, https://doi.org/10.1016/j.arth.2016.03.028, 2016.
Collins, G. S., Reitsma, J. B., Altman, D. G., and Moons, K. G.: Transparent reporting of a multivariable prediction model for individual prognosis or diagnosis (TRIPOD): the TRIPOD statement, BMC Med., 13, 1, https://doi.org/10.1186/s12916-014-0241-z, 2015.
Cook, J., Cook, E., Landsman, A. S., Basile, P., Dinh, T., Lyons, T., Rosenblum, B., and Giurini, J.: A retrospective assessment of partial calcanectomies and factors influencing postoperative course, J. Foot Ankle Surg., 46, 248–255, https://doi.org/10.1053/j.jfas.2007.03.016, 2007.
Diaz-Ledezma, C., Higuera, C. A., and Parvizi, J.: Success after treatment of periprosthetic joint infection: a Delphi-based international multidisciplinary consensus, Clin. Orthop. Relat. Res., 471, 2374–2382, https://doi.org/10.1007/s11999-013-2866-1, 2013.
Duffy, S. D., Ahearn, N., Darley, E. S., Porteous, A. J., Murray, J. R., and Howells, N. R.: Analysis Of The KLIC-score; An Outcome Predictor Tool For Prosthetic Joint Infections Treated With Debridement, Antibiotics And Implant Retention, J. Bone Joint Infect., 3, 150–155, https://doi.org/10.7150/jbji.21846, 2018.
Ensor, J., Martin, E. C., and Riley, R. D.: pmsampsize: Calculates the minimum sample size required for developing a multivariable prediction model, Version 1.0.2, available at: https://CRAN.R-project.org/package=pmsampsize (last access: June 2020), 2019.
Faschingbauer, M., Bieger, R., Kappe, T., Weiner, C., Freitag, T., and Reichel H.: Difficult to treat: are there organism-dependent differences and overall risk factors in success rates for two-stage knee revision?, Arch. Orthop. Trauma Surg., 140, 1595–1602, https://doi.org/10.1007/s00402-020-03335-4, 2020.
Ford, A. N., Holzmeister, A. M., Rees, H. W., and Belich, P. D.: Characterization of outcomes of 2-stage exchange arthroplasty in the treatment of prosthetic joint infections, J. Arthroplasty, 33, S224–S227, https://doi.org/10.1016/j.arth.2018.02.043, 2018.
Garcia Del Pozo, E., Collazos, J., Carton, J. A., Camporro, D., and Asensi, V.: Factors predictive of relapse in adult bacterial osteomyelitis of long bones, BMC Infect. Dis., 18, 635, https://doi.org/10.1186/s12879-018-3550-6, 2018.
Gordon, M. and Lumley, T.: Forestplot: advanced forest plot using “grid” graphics. Version 1.1, available at: https://cran.r-project.org/web/packages/forestplot/index.html, last access: June 2020.
Grant, S. W., Collins, G. S., and Nashef, S. A. M.: Statistical Primer: developing and validating a risk prediction model, Eur. J. Cardio-Thoracic Surg., 54, 203–208, https://doi.org/10.1093/ejcts/ezy180, 2018.
Grossi, O., Asseray, N., Bourigault, C., Corvec, S., Valette, M., Navas, D., Happi-Djeukou, L., Touchais, S., Bemer, P., and Boutoille, D.: Gram-negative prosthetic joint infections managed according to a multidisciplinary standardised approach: risk factors for failure and outcome with and without fluoroquinolones, J. Antimicrob. Chemother., 71, 2593–2597, https://doi.org/10.1093/jac/dkw202, 2016.
Hayden, J. A., van der Windt, D., Cartwright, J. L., Cote, P., and Bombardier, C.: Assessing bias in studies of prognostic factors, Ann. Intern. Med., 158, 280–286, https://doi.org/10.7326/0003-4819-158-4-201302190-00009, 2013.
Hoell, S., Sieweke, A., Gosheger, G., Hardes, J., Dieckmann, R., Ahrens, H., and Streitbuerger, A.: Eradication rates, risk factors, and implant selection in two-stage revision knee arthroplasty: a mid-term follow-up study, J. Orthop. Surg. Res., 11, 93, https://doi.org/10.1186/s13018-016-0428-4, 2016.
Hopkins, L., Brown-Broderick, J., Hearn, J., Malcolm, J., Chan, J., Hicks-Boucher, W., De Sousa, F., Walker, M. C., and Gagne, S.: Implementation of a referral to discharge glycaemic control initiative for reduction of surgical site infections in gynaecologic oncology patients, Gynecologic Oncology, 146, 228–233, https://doi.org/10.1016/j.ygyno.2017.05.021, 2017.
Hotchen, A. J., Dudareva, M., Ferguson, J. Y., Sendi, P., and McNally, M. A.: The BACH classification of long bone osteomyelitis, Bone Joint Res., 8, 459–468, https://doi.org/10.1302/2046-3758.810.BJR-2019-0050.R1, 2019.
Jhan, S. W., Lu, Y. D., Lee, M. S., Lee, C. H., Wang, J. W., and Kuo, F. C.: The risk factors of failed reimplantation arthroplasty for periprosthetic hip infection, BMC Musculoskel. Dis., 18, 255, https://doi.org/10.1186/s13018-016-0428-4, 2017.
Kandel, C. E., Jenkinson, R., Daneman, N., Hansen, B. E., Muller, M. P., Katz, K. C., Widdifield, J., Bogoch, E., Ward, S., Sajja, A., Garcia Jeldes, F., and McGeer, A.: Predictors of treatment failure for hip and knee prosthetic joint infections in the setting of 1- and 2-stage exchange arthroplasty: a multicenter retrospective cohort, Open Forum Infectious Diseases, 6, ofz452, https://doi.org/10.1093/ofid/ofz452, 2019.
Kheir, M. M., Tan, T. L., George, J., Higuera, C. A., Maltenfort, M. G., and Parvizi, J.: Development and evaluation of a prognostic calculator for the surgical treatment of periprosthetic joint infection, J. Arthroplasty, 33, 2986–2992, https://doi.org/10.1016/j.arth.2016.09.017, 2018.
Kurd, M. F. and Ghanem, E.: Two-stage exchange knee arthoplasty. Does resistance of the infecting organism influence the outcome?, Clin. Orthop. Relat. Res., 468, 2060–2066, https://doi.org/10.1007/s11999-010-1296-6, 2010.
Lam, A., Richardson, S. S., Buksbaum, J., Markowitz, J., Henry, M. W., Miller, A. O., Rozbruch, S. R., and Fragomen, A. T.: Chronic Osteomyelitis of the tibia and ankle treated with Limb Salvage Reconstruction, J. Bone Joint Infect., 4, 306–313, https://doi.org/10.7150/jbji.40337, 2019.
Lange, J., Troelsen, A., and Soballe, K.: Chronic periprosthetic hip joint infection. A retrospective, observational study on the treatment strategy and prognosis in 130 non-selected patients, PLOS One, 11, e0163457, https://doi.org/10.1371/journal.pone.0163457, 2016.
Lipsky, B. A., Berendt, A. R., Deery, H. G., Embil, J. M., Joseph, W. S., Karchmer, A. W., LeFrock, J., Lew, D. P., Mader, J. T., Norden, C., and Tan, J. S.: Diagnosis and treatment of diabetic foot infections, Clin. Infect. Dis., 39, 885–910, https://doi.org/10.1086/424846, 2004.
Ma, C. Y., Lu, Y. D., Bell, K. L., Wang, J. W., Ko, J. Y., Wang, C. J., and Kuo, F. C.: Predictors of treatment failure after 2-stage reimplantation for infected total knee arthroplasty: a 2- to 10-year follow-up, J. Arthroplasty, 33, 2234–2239, https://doi.org/10.1016/j.arth.2018.02.007, 2018.
Mader, J. T., Shirtliff, M., and Calhoun, J. H.: Staging and staging application in osteomyelitis, Clin. Infect. Dis., 25, 1303–1309, https://doi.org/10.1086/516149, 1997.
McPherson, E. J., Woodson, C., Holtom, P., Roidis, N., Shufelt, C., and Patzakis, M.: Periprosthetic total hip infection: outcomes using a staging system, Clin. Orthop. Relat. Res., 403, 8–15, 2002.
Merlet, A., Cazanave, C., Dauchy, F. A., Dutronc, H., Casoli, V., Chauveaux, D., De Barbeyrac, B., and Dupon, M.: Prognostic factors of calcaneal osteomyelitis, Scand. J. Infect. Dis., 46, 555–560, https://doi.org/10.3109/00365548.2014.914241, 2014.
Metsemakers, W. J., Morgenstern, M., McNally, M. A., Moriarty, T. F., McFadyen, I., Scarborough, M., Athanasou, N. A., Ochsner, P. E., Kuehl, R., Raschke, M., Borens, O., Xie, Z., Velkes, S., Hungerer, S., Kates, S. L., Zalavras, C., Giannoudis, P. V., Richards, R. G., and Verhofstad, M. H. J.: Fracture-related infection: a consensus on definition from an international expert group, Injury, 49, 505–510, 10.1016/j.injury.2017.08.040, 2018.
Moons, K. G., Altman, D. G., Reitsma, J. B., Ioannidis, J. P., Macaskill, P., Steyerberg, E. W., Vickers, A. J., Ransohoff, D. F., and Collins, G. S.: Transparent reporting of a multivariable prediction model for individual prognosis or diagnosis (TRIPOD): explanation and elaboration, Ann. Intern. Med., 162, W1–W73, https://doi.org/10.7326/m14-0698, 2015.
Moons, K. G. M., de Groot, J. A. H., Bouwmeester, W., Vergouwe, Y., Mallett, S., Altman, D. G., Reitsma, J. B., and Collins, G. S.: Critical appraisal and data extraction for systematic reviews of prediction modelling studies: the CHARMS checklist, PloS Med., 11, e1001744, https://doi.org/10.1371/journal.pmed.1001744, 2014.
Moore, A. J., Blom, A. W., Whitehouse, M. R., and Gooberman-Hill, R.: Deep prosthetic joint infection: a qualitative study of the impact on patients and their experiences of revision surgery, BMJ Open, 5, e0009495, https://doi.org/10.1136/bmjopen-2015-009495, 2015.
Mortazavi, S. M. J., Vegari, D., Ho, A., Zmistowski, B., and Parvizi, J.: Two-stage exchange arthroplasty for infected total knee arthroplasty: predictors of failure, Clin. Orthop. Relat. Res., 469, 3049–3054, https://doi.org/10.1007/s11999-011-2030-8, 2011.
National Institute for Health and Care Excellence: Lipid modification: cardiovascular risk assessment and the modification of blood lipids for the primary and secondary prevention of cardiovascular disease (NICE Clinical Guideline No. 181), available at: https://www.nice.org.uk/guidance/cg181 (last access: June 2020), 2016.
National Institute for Health and Care Excellence: Pneumonia in adults (NICE Quality Standard No. 110), available at: https://www.nice.org.uk/guidance/qs110 (last access: June 2020), 2016.
Norman, K., Pichard, C., Lochs, H., and Pirlich, M.: Prognostic impact of disease-related malnutrition, Clinical Nutrition, 27, 5–15, https://doi.org/10.1016/j.clnu.2007.10.007, 2008.
Parvizi, J. and Gehrke, T.: International Consensus Group on Periprosthetic Joint Infection, Definition of periprosthetic joint infection, J. Arthroplasty, 29, 1331, https://doi.org/10.1016/j.arth.2014.03.009, 2014.
Petis, S. M., Perry, K. I., Mabry, T. M., Hanssen, A. D., Berry, D. J., and Abdel, M. P.: Two-stage exchange protocol for periprosthetic joint infection following total knee arthroplasty in 245 knees without prior treatment for infection, J. Bone Joint Surg. Am., 101, 239–249, https://doi.org/10.2106/jbjs.17.01103, 2019.
R Core Team: R: A language and environment for statistical computing. R Foundation for Statistical Computing, Vienna, Austria, available at: https://www.R-project.org/, last access: June 2020.
Russell, C. D., Tsang, S. T. J., Simpson, A. H. R. W., and Sutherland, R. K.: Outcomes, microbiology and antimicrobial usage in pressure ulcer-related pelvic osteomyelitis, messages for clinical practice, J. Bone Joint Infect., 5, 67–75, 10.7150/jbji.41779, 2020.
Sabry, F. Y., Buller, L., Ahmed, S., Klika, A. K., and Barsoum, W. K.: Preoperative prediction of failure following two-stage revision for knee prosthetic joint infections, J. Arthroplasty, 29, 115–121, https://doi.org/10.1016/j.arth.2013.04.016, 2014.
Sakellariou, V. I., Poultsides, L. A., Vasilakakos, T., Sculco, P., Ma, Y., and Sculco, T. P.: Risk factors for recurrence of periprosthetic knee infection, J. Arthroplasty, 30, 1618-1622, https://doi.org/10.1016/j.arth.2015.04.005, 2015.
Schooling, C. M. and Jones, H. E.: Clarifying questions about “risk factors”: predictors versus explanation, Emerg. Themes Epidemiol., 15, 10, https://doi.org/10.1186/s12982-018-0080-z, 2018.
Son, M. S., Lau, E., Parvizi, J., Mont, M. A., Bozic, K. J., and Kurtz, S.: What are the frequency, associated factors, and mortality of amputation and arthrodesis after failed infected TKA?, Clin. Orthop. Relat. Res., 475, 2905–2913, https://doi.org/10.1007/s11999-017-5285-x, 2017.
Souza Jorge, L., Chueire, A. G., Silva Fucuta, P., Machado, M. N., Oliveira, M. G. L., Nakazone, M. A., and Salles, M. J.: Predisposing factors for recurrence of chronic posttraumatic osteomyelitis: a retrospective observational cohort study from a tertiary referral centre in Brazil, Patient Saf. Surg., 11, 17, https://doi.org/10.1186/s13037-017-0133-1, 2017.
Stiell, I. G., Greenberg, G. H., McKnight, R. D., Nair, R. C., McDowell, I., and Worthington, J. R.: A study to develop clinical decision rules for the use of radiography in acute ankle injuries, Ann. Emerg. Med., 21, 384–390, https://doi.org/10.1016/s0196-0644(05)82656-3, 1992.
Thomsen, T., Villebro, N., and Møller, A. M.: Interventions for preoperative smoking cessation, Cochrane Db. Syst. Rev., 3, CD002294, https://doi.org/10.1002/14651858.CD002294.pub4, 2014.
Trickett, R. W., Mudge, E., Price, P., and Pallister, I.: A qualitative approach to recovery after open tibial fracture: the road to a novel, patient-derived recovery scale, Injury, 43, 1071–1078, https://doi.org/10.1016/j.injury.2012.01.027, 2012.
Truntzer, J., Vopat, B., Feldstein, M., and Matityahu, A.: Smoking cessation and bone healing: optimal cessation timing, Eur. J. Orthop. Surg. Traumatol., 25, 211–215, https://doi.org/10.1007/s00590-014-1488-y, 2014.
Watts, C. D., Wagner, E. R., Houdek, M. T., Osmon, D. R., Hanssen, A. D., Lewallen, D. G., and Mabry, T. M.: Morbid obesity: a significant risk factor for failure of two-stage revision total knee arthroplasty for infection, J. Bone Joint Surg. Am., 96, e154, https://doi.org/10.2106/jbjs.m.01289, 2014.
Wang, Q., Goswami, K., Kuo, F. C., Xu, C., Tan, T. L., and Parvizi, J.: Two-stage exchange arthroplasty for periprosthetic joint infection: the rate and reason for the attrition after the first stage, J. Arthroplasty, 34, 2749–2756, https://doi.org/10.1016/j.arth.2019.06.021, 2019.
Wang, S. H., Xu, C., Tan, T. L., Goswami, K., Cooper, A. M., and Parvizi, J.: Increased postoperative glucose variability is associated with adverse outcome following two-stage exchange arthroplasty for periprosthetic joint infection, J. Arthroplasty, 35, 1368–1373, https://doi.org/10.1016/j.arth.2019.11.046, 2019.
Wishart, G. C., Azzato, E. M., Greenberg, D. C., Rashbass, J., Kearins, O., Lawrence, G., Caldas, C., Pharoah, P. D. P.: PREDICT: a new UK prognostic model that predicts survival following surgery for invasive breast cancer, Breast Cancer Res., 12, R1, https://doi.org/10.1186/bcr2464, 2010.
Wolff, R. F., Moons, K. G., Riley, R. D., Whiting, P. F., Westwood, M., Collins, G. S., Reitsma, J. B., Kleijnen, J., Mallett, S. and PROBAST Group: PROBAST: A tool to assess the risk of bias and applicability of prediction model studies, Ann. Intern. Med., 170, 51–58, https://doi.org/10.7326/M18-1376, 2019.
Wouthuyzen-Bakker, M., Sebillotte, M., Lomas, J., Taylor, A., Benavent Palomares, A., Murillo, O., Parvizi, J., Shohat, N., Cobo Reinoso, J., Escudero Sanchez, R., Fernandez-Sampedro, M., Senneville, E., Huotari, K., Barbero, J. M., Garcia-Canete, J., Lora-Tamayo, J., Ferrari, M. C., Vaznaisiene, D., Yusuf, E., Aboltins, C., Trebse, R., Salles, M. J., Benito, N., Vila, A., Del Toro, M. D., Kramer, T. S., Petersdorf, S., Diaz-Brito, V., Tufan, Z. K., Sanchez, M., Arvieux, C., Soriano, A. and ESGIAI Study Group: Clinical outcome and risk factors for failure in late acute prosthetic joint infections treated with debridement and implant retention, J. Infect., 78, 40–47, https://doi.org/10.1016/j.jinf.2018.07.014, 2019.