the Creative Commons Attribution 4.0 License.
the Creative Commons Attribution 4.0 License.
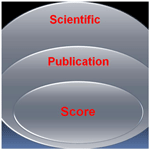
The scientific publication score – a new tool for summarizing evidence and data quality criteria of biomedical publications
Dieter Bettin
Thomas Maurer
Ferdinand Schlatt
Simon Bettin
The number of biomedical research articles increases by over 2.5 million publications each year, making it difficult to stay up to date. In this study, we introduce a standardized search and evaluation tool to combat this issue. Employing crowdsourcing, a large database of publications is gathered. Using a standardized data entry format, coined the “scientific publication score” (SPS), specific publication results can be easily aggregated, thereby allowing fast and accurate comparisons for clinical questions. The SPS combines two quality dimensions. The first captures the quality of evidence of the study using the evidence criteria defined by the Centre for Evidence-Based Medicine, Oxford, UK. The second is more fine-grained and considers the magnitude of statistical analyses on individual and specific results.
From 2014 to 2019, experts of the European Bone and Joint Infection Society (EBJIS) were asked to enter data of relevant publications about prosthetic joint infection. Data and evidence levels of specific results were averaged, summarized and ranked. A total of 366 publications were divided into two groups: (I) risk factors (e.g., host-related factors, pre- and postoperative issues) with 243 publications and (II) diagnostic methods (e.g., laboratory tests, imaging methods) with 123 publications.
After ranking, the highest score for risk factors of prosthetic joint infection were calculated by the SPS for anemia (mean 3.50± SD 0.91), malignancy (mean 3.17± SD 0.29) and previous alloarthroplasty (mean 3.00± SD 0.35). A comparison of the full SPS ranking with the ranking determined at the 2018 International Consensus Meeting (ICM) on Musculoskeletal Infection resulted in a Spearman rank correlation coefficient of 0.48 and a p value of 0.0382. The diagnostic methods ranked highest by the SPS were aspirate leucocyte count (mean 3.15± SD 1.21), interleukin 6 (mean 3.14± SD 1.07) and aspirate (neutrophils over 80 %) (mean 3.12± SD 0.63). The comparison to the ICM ranking yielded a Spearman rank correlation coefficient of 0.91 and a p value of 0.0015.
Our pilot study evaluated a new tool for the quality assessment of specific results based on the quality of the source publication. The SPS is suitable for a ranking of specific results by evidence and data quality criteria important for systematic reviews.
- Article
(581 KB) - Full-text XML
-
Supplement
(734 KB) - BibTeX
- EndNote
MEDLINE, the bibliographic database serving as the basis for PubMed, contains over 30 million publications in 30 000 journals. This content increases by 2.5 million publications each year. In biomedical and health informatics (BMHI), the information is doubled every 5 years (Hersh, 2020). Scientific results are sometimes individually presented and not always based on a concise format (Hersh, 2020; Poss et al., 2001). On this background, it is extremely difficult for medical professionals to stay informed.
Improvements were developed by the National Library of Medicine (NLM) by establishing an evidenced-based score for study quality (PubMed, 2022; Oxford Centre for Evidence-Based Medicine, 2009; Howick et al., 2011; Kittrie, 2018). The score is based on five evidence levels, from high-quality randomized trials (RCTs) with a score of 5 to a simple expert opinion with a score of 1.
While the evidence criteria are a good initial gauge of the evidence quality of a study, they give no insight into the actual individual and specific study results (e.g., intervention methods, outcome criteria, diagnostic methods and risk factors). Currently, specific study results are manually evaluated and aggregated in systematic reviews and meta-analyses (Bhandari et al., 2001). To expedite this aggregation process, we introduce the “scientific publication score” (SPS) for use in systematic reviews. It combines evidence quality criteria on the study and specific result level, giving insights into clinical questions.
Our study was organized in two parts: (1) the development of the definition criteria for the scientific publication score (SPS) and (2) the control of the SPS in an empirical pilot study evaluation.
2.1 Study level (evidence quality criteria)
The study level relies on the well-known evidence quality criteria, previously defined by the Centre for Evidence-Based Medicine, Oxford, UK. (Table 1; Oxford Centre for Evidence-Based Medicine, 2009; Howick et al., 2011). The study level is normally reported on the front page of articles in most high-impact journals. It summarizes the overall evidence quality of a study but ignores specific statistical results (e.g., when two interventions are being compared).
2.2 Data level (data quality criteria)
The data level is defined as a five-level score over several statistical criteria (see Table 2), similar to the ones used in alloplastic registers, clinical trials and meta-analyses (W-Dahl et al., 2021; Bhandari et al., 2001). Where applicable, the data level is recorded for each intervention of a study individually. Therefore, a study may have multiple data levels. If multiple criteria are determined for an intervention within a study, such as the number of participants and the sensitivity, the median level is used. While it is not an exhaustive list of possible statistical outcomes, nearly all studies determine at least one of the criteria. For an initial evaluation of the viability of the SPS, this list is therefore sufficient. With the experience gained from our pilot study, it will be extended in the future.
2.3 Calculation of the final score
The final score for an intervention is computed by averaging the study level and data level for a single recorded publication. In addition, the score is multiplied by the valence of the result, i.e., if the intervention was found to have a negative effect, the score is multiplied by −1. The overall score is then computed by averaging over all publications reporting on that intervention. Specifically, the SPS is defined as
for an intervention i, recorded publications Pi, study level (SL), data level (DL) and valence v.
2.4 Pilot study
We tested and evaluated the SPS using a crowdsourcing pilot study. The primary aim of the study was to evaluate whether the SPS can act as a lower-quality but lower-effort replacement in systematic reviews, especially for clinical questions lacking a comprehensive or up-to-date review. Experts from the field of bone and joint infections were tasked (on a voluntary basis) with inputting and rating publications using the SPS on an online platform. While this procedure lacks a defined search strategy and, thus, runs the risk of excluding a large amount of relevant publications, it was a cost-effective measure to obtain a large enough sample size to evaluate the validity of the SPS. To evaluate the validity, we compare the rankings of interventions and outcomes obtained from the expert scores with the rankings from the Consensus Meeting on Musculoskeletal Infection (Orthopedic Research Society, 2018).
A simple online platform was programmed. Example images are demonstrated in the Supplement. The basic characteristic of this platform was the ability to download publications from PubMed into different theme portals, such as prosthetic joint infection, basic science, bone regeneration after infection, bone tumor infection, diabetic foot infection, health economics, native joint infection, osteomyelitis, septic non-union, soft tissue infection, spondylitis, Gustilo Grade III open fracture and infection animal model (PubMed, 2022).
From 2014 to 2019, the (350) members of the European Bone and Joint Infection Society (EBJIS) were contacted by email and asked to propose clinical questions, link them to relevant publications and score them according to the SPS. The SPS program is organized into four sections:
-
The program begins with the Questions section and asks “Which are the highest risk factors for prosthetic joint infection (PJI)?” and “Which are the best diagnostic methods for prosthetic joint infection (PJI)?”.
-
The second section is Themes, which comprises the risk factors and diagnostic methods
-
The Categories section entails the prosthetic joint infection (PJI).
-
The Fields section comprises general infections.
2.5 Statistical analysis
The Spearman rank correlation test was calculated to compare the SPS results to the 2018 International Consensus Meeting on Musculoskeletal Infection results (Python SciPy stats package, version 1.6.1) (Orthopedic Research Society, 2018; Boyle et al., 2018).
A total of 488 publications were imported into the SPS program. The publications were not equally distributed among the topics. The largest two groups, risk factors and diagnostic methods, contained 243 publications and 123 publications, respectively. As multiple experts were able to score a single publication, a total of 722 scores across the 366 publications were considered in our analysis.
3.1 Pilot study group I: risk factors
The ranking results of the SPS and the International Consensus Meeting (ICM) identified similar risk factors for prosthetic joint infection (PJI) (Table 3).
The SPS revealed the highest numerical values for anemia (mean 3.5± SD 0.91), malignancy (mean 3.17± SD 0.29) and previous alloarthroplasty (mean 3.0± SD 0.35). Moreover, high SPS values were noted for previous joint surgery (mean 2.94± SD 1.26), wound healing problems (mean 2.74± SD 1.57), diabetes mellitus (mean 2.64± SD 1.46), poor vascular perfusion (mean 2.5± SD 1.8), malnutrition (mean 2.43± SD 0.79) and high body mass index (BMI; mean 2.33± SD 1.92). The ICM also identified these factors with a strong evidence strength (5 of 5 points).
A slight deviation was noted for previous alloarthroplasty (mean 3.0± SD 0.35) and immunosuppression (mean 2.27± SD 0.9), which were classified in ICM results with only moderate evidence strength (4 of 5 points).
Other SPS risk factors such as operation duration, surgical experience, persistent drainage, previous open fracture with remnants of implants, radiotherapy and operation room traffic were not addressed by the ICM results. This could be related to the fact that the ICM score is intended to have a better common agreement. Therefore, they voted only for a selected and lower number of criteria.
The differences between the risk factors were minimal with a large overlap of the standard deviations. This could be related to a lower number of publications for some risk factors (mean of 12.95 with a range of 3 to 53). Correlating only the risk factor scores found in both the final SPS and the ICM consensus meeting shows a moderate association, with a Spearman rank correlation coefficient of 0.48 (p=0.0385).
Table 3Pilot study group I: risk factors.
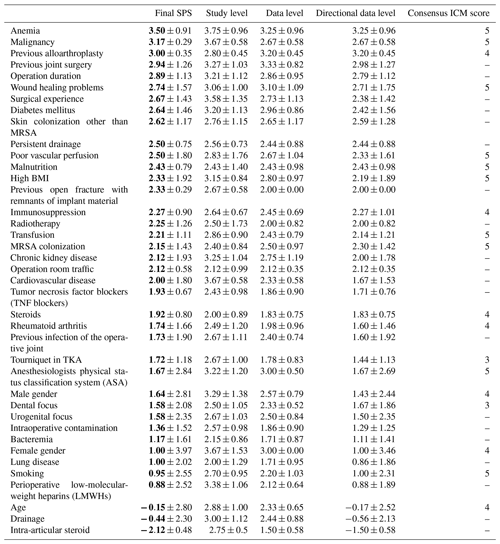
Data are represented as the mean ± SD, with bold font denoting the final SPS mean. The 2018 Consensus Meeting ICM score numbers represent the following: 5 – strong evidence strength, 4 – moderate evidence strength, 3 – limited evidence strength, 2 – low evidence strength and 1 – no evidence strength. MRSA denotes methicillin-resistant Staphylococcus aureus, and TKA represents total knee arthroplasty. “–” represents no consensus ICM score.
3.2 Pilot study group II: diagnostic methods
The SPS and the International Consensus Meeting (ICM) ranking results identified similar diagnostic methods for prosthetic joint infection (PJI) (Table 4).
The SPS revealed the highest numerical values for the aspirate leucocyte count (mean 3.15± SD 1.21), interleukin 6 (mean 3.14± SD 1.07) and aspirate (neutrophiles over 80 %) (mean 3.12± SD 0.63).
Furthermore high SPS values were noted for histology (mean 3.05± SD 0.69), sonication (mean 2.79± SD 0.64) and joint aspiration culture (mean 2.61± SD 1.05). The ICM also identified these factors with a moderate evidence strength (4 of 5 points).
The lowest SPS diagnose methods were noted for erythrocyte sedimentation rate (ESR; mean 0.57± SD 3.25) and white blood cells (mean 0.17± SD 3.16). The ICM also identified these factors with a low or no evidence strength (1–2 of 5 points).
Other SPS diagnostic methods such as leucocyte bone scan, TNF-alpha (tumor necrosis factor alpha), FDG PET (fluorodeoxyglucose positron emission tomography), combination of CRP (C-reactive protein) and IL-6 (interleukin 6), soluble intracellular adhesion molecules, and three-phase bone scan were not addressed by the ICM results.
Differences between the diagnostic methods were very small with large overlapping standard deviations. This could be related to a lower number of publications that evaluate diagnostic methods (mean of 10.75 with a range of 3 to 32). Correlating only the diagnostic method scores found in both the final SPS and the ICM consensus meeting shows a high association, with a Spearman rank correlation coefficient of 0.91 (p=0.0015).
Table 4Pilot study group II: diagnostic methods.
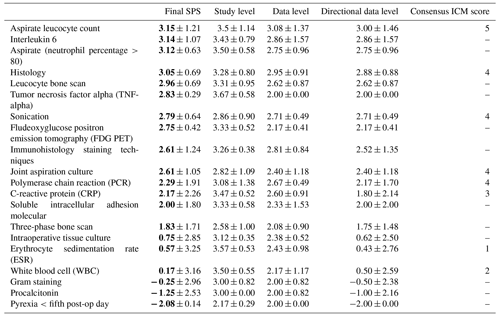
Data are represented as the mean ± SD, with bold font denoting the final SPS mean. The 2018 Consensus Meeting ICM score numbers represent the following: 5 – strong evidence strength, 4 – moderate evidence strength, 3 – limited evidence strength, 2 – low evidence strength and 1 – no evidence strength. “–” represents no consensus ICM score.
Our pilot study evaluated, for the first time, the quality of specific publication results by taking the quality of the source publication into account. The SPS program is able to organize the results in a standardized and concise format. The combination of evidence and data evaluation is a good instrument to summarize the quality of the specific results. The main value of the SPS is the possibility of one evaluation in the setting of the continuous publication of new papers in each subject group. The SPS offers a very quick incorporation of different analytic methods and outcome criteria in one score.
Conventional meta-analyses usually focus on a single outcome criteria and follow a rigorous quantitative methodology with the advantage of an increased study sample size, increased statistical power and, thus, improved quantitative estimates (Haidich, 2010). Systematic reviews similarly attempt to aggregate evidence but additionally handle study heterogeneity, co-influencing factors and other eligibility criteria (Ahn et al., 2018). The SPS, as such, functions as a middle ground between both. As it simply summarizes study results into a categorical score, it lacks mathematical rigor compared with meta-analyses, but it enables one to summarize over heterogeneous studies and evidence sources, which is important in systematic reviews (Haidich, 2010). In an empirical evaluation, the SPS achieves a moderate to high correlation with the results from the second International Consensus Meeting on Musculoskeletal Infection (Orthopedic Research Society, 2018). Furthermore, due to its simplicity, the SPS is easily scalable. Extracted scores from a publication can be integrated into a database, and the aggregated results for a clinical question are automatically updated. We take advantage of this concise format in our initial pilot study and intend to automate the extraction process in the future.
The highest scores for risk factors were noted for anemia, malignancy and previous alloarthroplasty. For diagnostic methods, the highest scores were obtained for aspirate leucocyte count, interleukin-6 level and aspirate (neutrophils over 80 %). The ranking does not comprehensively reflect the present clinical experience with respect to the importance of all possible risk factors. In particular, for persistent drainage, bacteraemia and intraoperative contamination as well as for the diagnostic method joint aspiration, some clinical deviations should be considered. Those factors might have higher scores considering their clinical relevance and the relatively low number of publications included in this study (mean of 10.75 for each criterion). Furthermore, for the risk factors, we noted some apparent outliers. For example, female gender and smoking had lower scores than the ICM. As explained above, these factors might have a higher score considering the mean of 12.95 publication for each criterion. A more extensive automatic screening procedure – including all ongoing publications – would certainly enhance the accuracy.
The obtained ranking results are similar and comparable to the results presented at the 2018 second International Consensus Meeting (ICM) on Musculoskeletal Infection (Orthopedic Research Society, 2018; Boyle et al., 2018). At this consensus conference, over 3500 publications were screened by 869 experts. However, the ICM is based on a very extensive and time-consuming procedure using the Delphi method for evaluation (Boyle et al., 2018). Experts gave answers to a list of specific questions in several rounds. The aggregated answers were discussed in between rounds, and individual answers could be revised in subsequent rounds, taking the answers of other experts into account. Using this Delphi method, the whole group approximated the “correct answer” in the final scores.
4.1 Quality assessments
Quality assessments were frequently based solely on reviews using evidence-based criteria presented by the Cochrane Library or the UpToDate Database internet platform (Cochrance Library, 2016; Higgins et al., 2022; UpToDate, 2022). Those groups evaluate the evidence quality by considering randomization and blinding processes, population heterogeneity, indirectness and imprecision of results, and the publication bias, and they summarized these using the Grading of Recommendations, Assessment, Development and Evaluation (GRADE) system score noting a high, moderate, low or very low quality (Guyatt et al., 2011a, b). The main disadvantage of those approaches is their extensive and time-consuming nature. In the UpToDate Database, over 6800 highly specialized scientists are involved in keeping up with the review evaluation procedure (UpToDate, 2022).
4.2 Novelty
Our approach to include both evidence-based criteria and statistical data for specific publication results simultaneously is new. To our knowledge, no other group has done this before. Some authors have included additional information on patient numbers and follow-up criteria in their evidence classification (Trip Database-Blog, 2018; Trip Medical Database, 2022), but our approach includes a broader and more heterogeneous set of statistical measures. Assigning levels of evidence, including power analysis and follow-up criteria for orthopedic patients, for a similar research question has also been applied by Wright (2007).
More extensive subclassifications are possible with the SPS, which are needed to identify the most influential factors. Some authors have recommended the PICO (patient, intervention, comparison, outcome) method for quality assessments (UC Library Guides, 2022; Zhang et al., 2020). Using this approach, two or more interventions can be compared side by side with respect to their results of defined outcome criteria (Huang et al., 2006). Therefore, we used this method in an advanced form to address each clinical question in more detail by simultaneously ranking various diagnosis methods and risk factors. The modified Coleman methodology score (MCMS) is also applied to evaluate research methodology by advanced quality criteria (Table 5; Coleman et al., 2000).
The subsections of the MCMS are based on the subsections of the Consolidated Standards of Reporting Trials (CONSORT) statement (for randomized controlled trials) (Moher et al., 2012). A total score of 100 indicates that the study largely avoids chance, various biases and confounding factors (Coleman et al., 2000; Longo et al., 2015). One disadvantage of the MCMS is that it can only be used for surgical interventions. However, some information required for the diagnostic certainty, the procedure for assessing outcomes and the description of the subject selection process is not uniformly given in every publication. Therefore, the MCMS cannot be used as a framework for encoding evidence. The statistical results for the SPS are easier to identify. One advantage of the MCMS is the inclusion of selection and bias criteria (Boyle et al., 2018). The SPS can cover this by choosing the next lower level in the SPS voting.
4.3 Limitations
Our pilot study started with the idea that a large scientific community will answer open questions more easily. However, experts of the EBJIS were not able to evaluate all ongoing publications.
Additionally, experts may be biased to include specific publications for clinical questions that they deem relevant. Thus, our pilot study did not list all possible risk factors and diagnostic methods. Moreover, a publication bias had to be considered, as studies with negative results are more likely to remain unpublished (Poss et al., 2001). Furthermore, the voting bias could be influential, as users do not like to vote on lower study or data levels (Bhandari et al., 2001). Ethically, it is not easy to vote on other experts' publications or on one's own publications. An anonymous voting procedure addressed this limitation. All experts were further able to delete and edit their votes. The influence of “voting on one's own publications” might be diminished if other experts confirmed the voting result.
The rounding up and down of data levels resulted in considerable variations, reflected by the large standard deviations and the low correlation coefficient, especially in the risk factor group. Our results could also be influenced by individual preferences, leading to some user disagreements. However, this problem was also evident in the consensus meeting results, showing multiple nonunanimous decisions among the experts (Orthopedic Research Society, 2018; Boyle et al., 2018; Howick et al., 2011).
4.4 Future development
Potential improvements can be made by focusing on controlled studies (study level 1–3) with a high study and data level. Furthermore, a more rigorous training of experts in data entry could increase inter-rater agreement. Finally, more publications and ratings would result in a broader coverage of clinical categories and more conclusive results.
Further development potential lies in the (semi-)automation of evidence scoring and aggregation (Del Fiol et al., 2018). Several companies and research institutes are working on automating the meta-analysis process. Approaches range from aiding in evidence extraction from full texts to automatically aggregating study results over multiple publications or extracting claims from biomedical abstracts (Pradhan et al., 2019; Achakulvisut et al., 2020; Trip Database-Blog, 2022; Kittrie, 2018). Most approaches lack a framework for encoding evidence in a comprehensive and easy-to-aggregate form. We propose that SPS provides exactly this kind of framework and are actively investigating how to integrate it into an automated process as a search and seal of approval tool.
Our pilot study evaluated a new tool for the quality assessment of specific results in different publication qualities. SPS is suitable for ranking specific publication results by evidence and data quality criteria. However, the crowdsourcing methodology was unable to keep up with newly published publications. Modern methods of automatic data mining are expected to improve the coverage of search efficiency and quality verification in the future.
All data generated and analyzed during this study are included in this published article and are available from the corresponding author upon reasonable request.
The supplement related to this article is available online at: https://doi.org/10.5194/jbji-7-269-2022-supplement.
DB created the scientific publication score (SPS) and was responsible for the conceptualization, study design and supervision of the pilot study; he also wrote the original draft of the paper. TM programmed the pilot version of the internet platform to organize the data collection. FS conducted the formal analysis and performed data analysis based on his data mining and statistical expertise. SB supported the SPS idea and reviewed and edited the manuscript for the final approval.
At least one of the (co-)authors is a member of the editorial board of Journal of Bone and Joint Infection. The peer-review process was guided by an independent editor, and the authors also have no other competing interests to declare.
The scientific publications evaluated have been carried out in accordance with the ethical standards of each included study and the principles of the Declaration of Helsinki.
Publisher's note: Copernicus Publications remains neutral with regard to jurisdictional claims in published maps and institutional affiliations.
The authors would like to thank the members of the EBJIS for supporting the pilot study. We also thank Leonie Bettin for her support of the SPS idea, the careful control reading, language customization, and formal ideas to improve tables and data presentation. We acknowledge support from the Open Access Publication Fund of the University of Münster.
This paper was edited by Rihard Trebse and reviewed by six anonymous referees.
Achakulvisut, T., Bhagavatula, C., Acuna, D., and Kording, K.: Claim Extraction in Biomedical Publications using Deep Discourse Model and Transfer Learning. arXiv [preprint], arXiv:1907.00962, https://doi.org/10.48550/arXiv.1907.00962, 2020.
Ahn, E., Kahn, H.: Introduction to systemic review and meta-analysis, Korean J.Anesthesiol., 71, 103–112, https://doi.org/10.4097/kjae.2018.71.2.103, 2018.
Bhandari, M., Morrow, F., Kulkarni, A. V., and Tornetta, P.: Meta-analyses in orthopaedic surgery. A systematic review of their methodologies, J. Bone Joint Am., 83, 15–24, 2001.
Boyle, K. K., Kuo, F.-C., Horcajada, J. P., Hughes, H., Cavagnaro, L., Marculescu, C., McLaren, A., Nodzo, S. R., Riccio, G., Sendi, P., Silibovsky, R., Stammers, J., Tan, T. L., and Wimmer, M.: General Assembly, Treatment, Antimicrobials: Proceedings of International Consensus on Orthopedic Infections, J. Arthroplasty, 34, 225–237, https://doi.org/10.1016/j.arth.2018.09.074, 2018.
Cochrance Library: https://www.cochranelibrary.com/advanced-search (last access: 20 August 2022), Wiley, © 2000–2022, 2016.
Coleman, B. D., Khan, K. M., Maffulli, N., Cook, J. L., and Wark, J. D.: Studies of surgical outcome after patellar tendino pathy: Clinical significance of methodological deficiencies and guidelines for future studies. Victorian Institute of Sport Tendon Study Group, Scand. J. Med. Sci. Sports, 10, 2–11, https://doi.org/10.1034/j.1600-0838.2000.010001002.x, 2000.
Del Fiol, G., Michelson, M., Iorio, A., Cotoi, C., and Haynes, R. B.: A Deep Learning Method to Automatically Identify Reports of Scientifically Rigorous Clinical Research from the Biomedical Literature: Comparative Analytic Study, J. Med. Internet Res., 20, e10281, https://doi.org/10.2196/10281, 2018.
Guyatt, G., Oxman, A. D., Akl, E. A., Kunz, R., Vist, G., Brozek, J., Norris, S., Falck-Ytter, Y., Glasziou, P., DeBeer, H., Jaeschke, R., Rind, D., Meerpohl, J., Dahm, P., and Schünemann, H. J.: GRADE guidelines: 1. Introduction-GRADE evidence profiles and summary of findings tables, J. Clin. Epidemiol., 64, 383–394, https://doi.org/10.1016/j.jclinepi.2010.04.026, 2011a.
Guyatt, G. H., Oxman, A. D., Vist, G., Kunz, R., Brozek, J., Alonso-Coello, P., Montori, V., Akl, E. A., Djulbegovic, B., Falck-Ytter, Y., Norris, S. L., Williams, J. W., Atkins, D., Meerpohl, J., and Schünemann, H. J.: GRADE guidelines: 4. Rating the quality of evidence–study limitations (risk of bias), J. Clin. Epidemiol., 64, 407–415, https://doi.org/10.1016/j.jclinepi.2010.07.017, 2011b.
Haidich, A. B.: Meta-analysis in medical research, Hippokratia, 2010, 29–37, 2010.
Hersh, W.: Information Retrieval: A Biomedical and Health Perspective, Fourth Edition, Springer Health Informatics 2020, Oregon Health & Science University, Portland, OR, USA, https://doi.org/10.1007/978-3-030-47686-1, 2020.
Higgins, J. P. T., Thomas, J., Chandler, J., Cumpston, M., Li, T., Page, M. J., and Welch, V. A. (Eds.): Cochrane Handbook for Systematic Reviews of Interventions version 6.3 (updated February 2022), Cochrane, 2022, http://www.training.cochrane.org/handbook last access 20 August 2022.
Howick, J., Chalmers, I., Glasziou, P., Greenhalgh, T., Heneghan, C., Liberati, A., Moschetti, I., Phillips, B., and Thornton, H.: The 2011 Oxford CEBM Levels of Evidence (Introductory Document), Oxford Centre for Evidence-Based Medicine, https://www.cebm.ox.ac.uk/resources/levels-of-evidence/ocebm-levels-of-evidence (last access: 22 August 2022), 2011.
Huang, X., Lin, J., and Demner-Fushman, D.: Evaluation of PICO as a knowledge representation for clinical questions, AMIA Annu. AMIA Symposium, 359–363, PMCID 17238363 PMC 1839740, 2006.
Kittrie, E.: The US National Library of Medicine: A Platform for Biomedical Discovery & Data-Powered Health. HT '18: Proceedings of the 29th on Hypertext and Social Media. HT '18: 29th ACM Conference on Hypertext and Social Media. Baltimore MD USA, 9–12 July 2018, 155 pp., https://doi.org/10.1145/3209542.3209546, 2018.
Longo, U. G., Rizzello, G., Loppini, M., Locher, J., Buchmann, S., Maffulli, N., and Denaro, V.: Multidirectional Instability of the Shoulder: A Systematic Review, Arthrosc. J. Arthrosc. Rel. Surg., 31, 2431–2443, https://doi.org/10.1016/j.arthro.2015.06.006, 2015.
Moher, D., Hopewell, S., Schulz, K. F., Schulz, K. F., Montori, V., Gøtzsche, P. C., Devereaux, P. J., Elbourne, D., Egger, M., and Altman, D. G.: CONSORT: CONSORT 2010 explanation and elaboration: updated guidelines for reporting parallel group randomised trials, Int. J. Surg., 10, 28–55, https://doi.org/10.1016/j.ijsu.2011.10.001, 2012.
Orthopedic Research Society: 2018 International Consensus on Musculoskeletal Infection- General Assembly: https://www.ors.org/icm-2018-general-assembly/, last access: 20 August 2022.
Oxford Centre for Evidence-Based Medicine: Levels of Evidence (March 2009): https://www.cebm.ox.ac.uk/resources/levels-of-evidence/oxford-centre-for-evidence-based-medicine-levels-of-evidence-march-2009/e/, last access: 20 August 2022.
Poss, R., Clark, C. R., and Heckman, J. D.: A Concise Format for Reporting the Longer-Term Follow-up Status of Patients Managed with Total Hip Arthroplasty, J. Bone Joint Surg. Am., 83, 1779–1780, https://doi.org/10.2106/00004623-200112000-00001, 2001.
Pradhan, R., Hoaglin, D. C., Cornell, M., Liu, W., Wang, V., and Yu, H.: Automatic extraction of quantitative data from ClinicalTrials.gov to conduct meta-analyses, J. Clin. Epidemiol., 105, 92–100, https://doi.org/10.1016/j.jclinepi.2018.08.023, 2019.
PubMed Clinical Query: National Library of Medicine NIH National Center for Biotechnology Information, Pub med.gov., https://pubmed.ncbi.nlm.nih.gov/clinical/, last access: 20 August 2022.
Trip Database-Blog: https://blog.tripdatabase.com/2018/06/07/automated-review-system-explained/, last access: 20 August 2022.
Trip Medical Database: https://tripdatabase.com, last access: 20 August 2022.
UC Library Guides: Evidence-Base Practice in Health University of Canberra Library, https://canberra.libguides.com/c.php?g=599346&p=4149722:, last access: 20 August 2022.
UpToDate Evidence-based Decision Support: https://www.wolterskluwer.com/en-nz/solutions/uptodate, last access: 20 August 2022.
W-Dahl, A., Kärrholm, J., Rogmark, C., Naucler, E., Natman, J., Bülow, E., Mohaddes, M., Sundberg, M., and Rolfson, O.: Annual Report 2021, SAR Swedish Arthroplasty Register, https://registercentrum.blob.core.windows.net/slr/r/SAR-Annual-Report-2021-SJlAFmlRl5.pdf, last access: 20 August 2022.
Wright, J. G.: A practical guide to assigning levels of evidence, J. Bone Joint Surg. Am., 89, 1128–1130, https://doi.org/10.2106/JBJS.F.01380, 2007.
Zhang, X., Geng, P., Zhang, T., Lu, Q., Gao, P., and Mei, J.: Aceso: PICO-guided Evidence Summarization on Medical Literature, IEEE J. Biomed. Inform., 24, 2663–2670, https://doi.org/10.1109/JBHI.2020.2984704, 2020.